Probabilistic population forecasts for Australia
New 2024-based population forecasts for Australia have been prepared, and a summary is available in the report here. The forecasts are probabilistic, and therefore include estimates of forecast uncertainty. They indicate that the population of 27.2 million in 2024 is likely to grow to between 29.3 and 32.3 million by 2034, and to between 30.7 and 37.9 million by 2044 (80% uncertainty range). A summary of forecast assumptions and an overview of the new probabilistic forecasting model are given in the report.
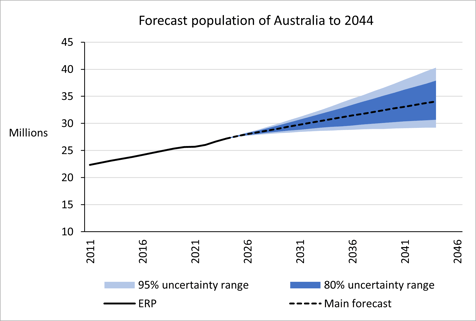
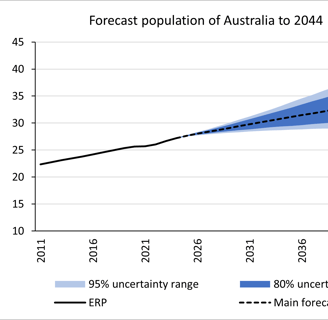
Frequently asked questions about probabilistic population forecasts
What is a probabilistic population forecast?
A probabilistic forecast consists of a distribution of possible future populations. It is created by running thousands of forecasts with randomly varying fertility, mortality and migration rates. The resulting population forecasts are then sorted in size order to give a distribution of possible future populations.
A probabilistic forecast is often created on the assumption that demographic processes will remain as variable and random in the future as they were in the past.
Almost all population forecasts inevitably contain some degree of error. Probabilistic forecasts acknowledge that future populations cannot be forecast precisely. Importantly, they offer information to users about the extent of the uncertainty. This varies over time, between age groups, between births, deaths, and migration, and between different populations. See, for example, the uncertainty ranges shown in the graphs in the report here.
How do I use probabilistic forecasts?
In using probabilistic forecasts, it is recommended that you first take the principal (middle) forecast and apply this in your analysis, discussions, and modelling, etc.
Then apply populations at 10% and 90% of the population distribution in your analysis/discussions/modelling to see if decisions would change. This covers the middle 80% of possible population futures. If you think decisions might change, then:
maybe delay the decision; or
make a decision which relates to the less uncertain period over the immediate future; or
make a decision that allows flexibility to respond to a range of population sizes later on.
You could instead use populations closer to the ends of the population distribution (such as 2.5% and 97.5%) to give a wider coverage of possible population futures. But the 10th and 90th percentiles should suffice for most purposes.
If the uncertainty range is wide, does that mean the forecast will be very wrong?
No, not necessarily. It means there is a wide range of possible future values that future population (or the demographic variable in question) could take. The forecast might turn out to be quite accurate, but it is also possible that it will have a high error.
What are the advantages and disadvantages of probabilistic population forecasts?
Advantages
Probabilistic forecasts clearly warn users that the demographic future is uncertain.
They also quantify the extent of forecast uncertainty, and demonstrate how this varies over time, across age groups, and between demographic components (births, deaths, migration).
The uncertainty ranges from probabilistic forecasts can be helpful for decisions-makers making risk asessments in planning, budgeting, investment, and policy decisions.
Probabilistic forecasts are created by statistical models which mimic the variability and randomness of demographic processes.
In the Advanced Demnographic Modelling probabilistic model, small area sampling variability is also modelled, which is important in forecasting small area populations.
Disadvantages
Probabilistic forecasts are more complicated to prepare than regular (deterministic) forecasts.
Probabilistic forecasts take much more computing time to calculate.
Every time a probabilistic forecast is run, there will be very small differences in the forecasts.
There is no absolute guarantee that future populations will lie within the forecast range. Huge global economic shocks, pandemics, wars, political upheaval, or major local disasters could result in actual future population numbers which fall outside the range of the forecast distribution.
Why wouldn’t I just use high and low scenario projections?
Unfortunately, high and low scenario projections contain serious problems for estimating forecast uncertainty. These scenarios are usually created by adjusting the projection assumptions from the main forecast by arbitrary amounts, such as 10%. It is almost impossible to judge how likely these scenarios are, or what the scenarios actually mean.
High and low scenarios often involve higher and lower fertility and migration assumptions, but not always variations in mortality. Whenever this is the case, future numbers of deaths, and the elderly population, will therefore vary much between them.
High and low scenarios for internal migration are difficult to apply because net internal migration must sum across areas to zero. If particular areas are allocated higher net internal migration gains than the principal forecast, then other areas must have lower net internal migration. This scenario would involve higher net internal migration than the main forecast for some areas, but lower net internal migration for others.
Most importantly, the range between high and low scenario projections does not provide reliable information about forecast uncertainty. First, these ranges do not come with any information about the likelihood of future population lying within the range. Second, a comparison with probabilistic forecasts would reveal that the high-low range for total population covers a specific percentage of likely future populations 5 years into the forecasts, but different percentages further into the future. The high-low range for the future numbers of births will cover a specific percentage of likely future numbers after 10 years, but for deaths the high-low range will cover a different percentage. The same applies to different age groups. High-low ranges are probabilistically inconsistent over time and between demographic variables. For more on this, see this paper by Nico Keilman and colleagues.
This isn’t to say that alternative population scenarios can’t be useful for specific purposes. But high and low scenarios cannot provide reliable uncertainty information.
Where can I find out more about probabilistic forecasts?
Suggested readings:
Keilman, N (2018) Probabilistic demographic forecasts. Vienna Yearbook of Population Research 2018 pp. 25-25.
National Research Council (2000) The uncertainty of population forecasts. Chapter 7 in Beyond Six Billion: Forecasting the World's Population. Washington, DC: The National Academies Press.
Keilman, N (2020) Uncertainty in population forecasts for the twenty-first century. Annual Review of Resource Economics 12 pp. 449-470.
Bijak, J. et al. (2015) Probabilistic population forecasts for informed decision making. Journal of Official Statistics 31(4) pp. 537-544.